Probability Distribution
The probability distribution allows the possibility of each outcome of a random experiment. Provides the possibility of various possible occurrences. Here you will learn more about probability distribution.
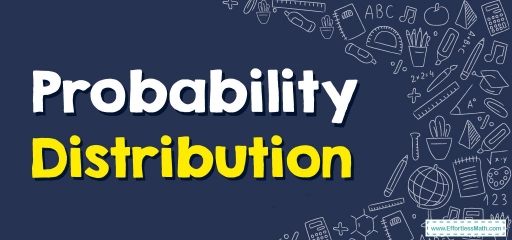
The probability distribution is one of the most important concepts in statistics. It has huge applications in trade, engineering, medicine, and other major sectors. It is mainly used for future predictions based on a sample for a random experiment.
Related Topics
A step-by-step guide to the probability distribution
The probability distribution gives the probable results for each random event. It is also defined based on the underlying sample space as a set of possible outcomes of each randomized experiment. These settings can be a set of real numbers or a set of vectors or a set of any entity. It is a part of probability and statistics.
Random experiments are defined as experimental results whose results are unpredictable. Suppose, if we toss a coin, we can not predict what outcome it will appear with either it will come as a Head or as a Tail.
The probable result of a random experiment is called an outcome. And a set of outcomes is called a sample point.
With the help of these experiments or events, we can always create a probability pattern table in terms of variables and probabilities.
Probability distribution of random variables
A random variable has a probability distribution that specifies the probability of its unknown values. Random variables can be discrete (not constant) or continuous, or both. That means it takes any of a designated finite or countable list of values, provided with a probability mass function feature of the random variable’s probability distribution, or can take any numerical value in an interval or set of intervals.
Two random variables with equal probability distribution can yet be different in their relationships with other random variables or whether they are independent of these.
Detecting a random variable means that the outcomes of randomly selecting values based on the variable probability distribution function are called random variables.
Types of the probability distribution
There are two types of probability distributions that are used for different purposes and different types of data production processes.
- Normal or cumulative probability distribution
- Binomial or discrete probability distribution
Cumulative probability distribution:
Cumulative probability distributions are also known as continuous probability distributions. In this distribution, a set of possible outcomes can take values in a continuous range. For example, a set of real numbers, is a continuous or normal distribution, as it gives all the possible outcomes of real numbers.
The formula for the normal distribution is:
\(\color{blue}{P\left(x\right)=\frac{1}{\sigma \sqrt{2\pi }}e^{-\frac{1}{2}\left(\frac{x-\mu }{\sigma }\right)^2}}\)
Where,
- \(\mu =\) Mean value
- \(σ =\) Standard distribution of probability
- \(x =\) Normal random variable
If mean \((μ) = 0\) and standard deviation \((σ) = 1\), then this distribution is known to be a normal distribution.
Discrete probability distribution:
A distribution is called a discrete probability distribution, in which a set of outcomes is discrete in nature. For example, if a dice is rolled, then all the possible outcomes are discrete and give a mass of outcomes. It is also known as the probability mass function.
Therefore, the outcomes of a binomial distribution include n repeated experiments, and the outcome may or may not occur. The formula for the binomial distribution is:
\(\color{blue}{P\left(x\right)=\frac{n!}{r!\left(n-r\right)!}.p^r\left(1-p\right)^{n-r}}\)
\(\color{blue}{P\left(x\right)=C\:\left(n,\:r\right).p^r\left(1-p\right)^{n-r}}\)
Where,
- \(n =\) Total number of events
- \(r =\) Total number of successful events
- \(p =\) Success on a single trial probability
- \(^nC_r=\left[\frac{n!}{r!\left(n-r\right)!}\right]\)
- \(1 – p =\) Failure Probability
Related to This Article
More math articles
- Top 10 CHSPE Math Prep Books (Our 2023 Favorite Picks)
- The Ultimate PARCC Algebra 1 Course (+FREE Worksheets)
- 5 Best Laptops For Teachers
- Which Test Should You Take: GED, TASC, or HiSET?
- Place Values Relationship
- How to Multiply two Binomials Using Algebra Tiles?
- How to Find The Slope of Roots: Derivative of Radicals
- 5th Grade MCAS Math FREE Sample Practice Questions
- Special Right Triangles
- 5th Grade SBAC Math FREE Sample Practice Questions
What people say about "Probability Distribution - Effortless Math: We Help Students Learn to LOVE Mathematics"?
No one replied yet.