Mastering the Metrics of Chance: A Complete Guide to Understanding Random Variables
Understanding random variables is pivotal in the fields of probability and statistics, as they provide a formal mechanism for quantifying the outcomes of random processes. Here’s a step-by-step guide to understanding random variables.
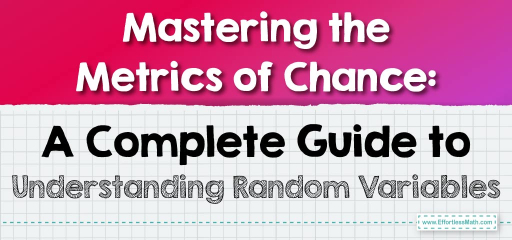
Step-by-step Guide to Understanding Random Variables
Here is a step-by-step guide to understanding random variables:
Step 1: Grasp the Concept of a Random Variable
- A random variable (RV) is a function that assigns a real number to each outcome in the sample space of a random experiment. Imagine flipping a coin: the outcome can be Heads or Tails, and we can assign the number \(0\) to Tails and \(1\) to Heads. Here, the outcomes have been quantified, which is exactly what a random variable does.
Step 2: Differentiate Between Discrete and Continuous Random Variables
- Discrete Random Variables: These have a countable number of possible values. For instance, the number of heads in ten coin flips is discrete because it can be any integer from \(0\) to \(10\).
- Continuous Random Variables: These can take on any value in a continuous range. The exact time it takes for a dropped ball to hit the ground would be modeled by a continuous random variable since time can be measured with infinite precision within a range.
Step 3: Probability Mass Function (PMF) for Discrete RVs
- The PMF is a function that gives the probability that a discrete random variable is exactly equal to some value. For example, in a single die roll, the PMF would give a probability of \(\frac{1}{6}\) for each outcome from \(1\) to \(6\).
Step 4: Probability Density Function (PDF) for Continuous RVs
- The PDF, conversely, is used for continuous random variables and represents the probability of the variable falling within a particular range of values. The probability for a specific point is not defined; instead, we look at intervals. For instance, if \(X\) is the time taken for a chemical reaction to occur, \(f(x)\) might give the density of probability across time intervals.
Step 5: Calculate Probabilities Using PMF and PDF
- For a discrete RV, you can find the probability of a particular outcome by simply plugging the value into the PMF.
- For a continuous RV, you find the probability of it falling within an interval by integrating the PDF over that interval. For example, to find the probability that \(X\) falls between \(a\) and \(b\), you would calculate \({∫_a}^{b}f(x) \ dx\).
Step 6: Notation and Terminology
- RVs are typically denoted by capital letters, such as \(X\), \(Y\), or \(Z\).
- The specific values that an RV can take are often denoted by lowercase letters like \(x\), \(y\), or \(z\).
Step 7: Expectation and Variance of RVs
- The expectation (or mean) of an RV is the long-run average value it takes on. It’s computed differently for discrete and continuous variables but represents the central tendency in both cases.
- The variance measures how much the values of the RV spread out from the mean. It gives an idea of the variability in the possible values of the RV.
Step 8: Applications of RVs
- In practice, RVs are used for modeling in various contexts, from simple games of chance to complex phenomena in finance, engineering, and science.
- For instance, discrete RVs can model the number of customers arriving at a store, while continuous RVs can model the fluctuations in stock prices.
Step 9: Practical Examples and Problem-Solving
- Engage with examples that apply both discrete and continuous RVs to solidify your understanding. Work on problems like calculating the expected number of heads in a series of coin flips or the probability that a bus arrives within five minutes.
Step 10: Advanced Concepts
- Once comfortable with the basics, delve into joint distributions, conditional probabilities, and the Central Limit Theorem, which are all underpinned by the concept of random variables.
By mastering the concept of random variables, you equip yourself with a critical tool for analyzing and making decisions based on uncertain events. This knowledge is not just academic; it is crucial for data science, risk management, and various fields that involve stochastic processes.
Related to This Article
More math articles
- TASC Math Formulas
- How to Write Linear Functions from Tables
- How to Solve the Absolute Value of Rational Numbers?
- What Skills Do I Need for the ASVAB Math Subtests?
- How to Prepare for the SSAT Middle-Level Math Test?
- How to Represente Proportional Relationships with Equations
- How to Master the Road to Achievement: “Praxis Core Math for Beginners” Comprehensive Answer Guide”
- How to Prepare for the HSPT Math Test?
- Unlocking the Secrets of Similar Polygons: Shape, Size, and Proportions!
- What’s The Best Laptop For Online Math Teachers?
What people say about "Mastering the Metrics of Chance: A Complete Guide to Understanding Random Variables - Effortless Math: We Help Students Learn to LOVE Mathematics"?
No one replied yet.